Ian Tenney
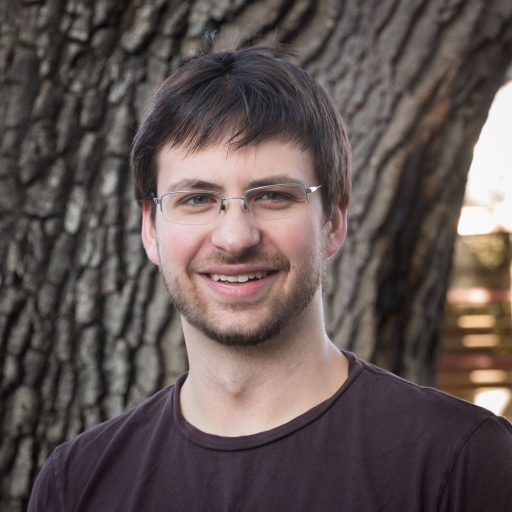
I am a Staff Research Scientist on the People + AI Research (PAIR) team at Google DeepMind. My group focuses on interpretability for large langauge models (LLMs), including visualization tools, attribution methods, and intrinsic analysis (a.k.a. BERTology) of model representations. Through these, we aim to answer questions like:
- Why did a model make this particular prediction?
- What kind of knowledge is stored in the parameters, and how is it represented and reasoned about?
- How can we build our own mental models of how - and when - AI works?
Among other things, I am a co-creator of the Learning Interpretability Tool (LIT) and author of BERT Rediscovers the Classical NLP Pipeline.
Previously, I’ve taught an NLP course at UC Berkeley School of Information. In a past life I was a physicist, studying ultrafast molecular and optical physics in the lab of Philip H. Bucksbaum at Stanford / SLAC.
When I’m not behind a computer I enjoy hiking and photography; you can find some of it here.
Contact: "if" + lastname + "@gmail.com"
(or @google.com
)
news
Jan 22, 2025 | Pleased to share that our recent TDA work was accepted to ICLR 2025! Check out the preprint here: Scalable Influence and Fact Tracing for Large Language Model Pretraining (Chang et al. 2025) |
---|---|
Dec 13, 2024 | New blog post & preprint on Scaling Training Data Attribution to understand what data an LLM learned from during open-domain pretraining. We’ve also released the dataset along with a web-based demo to explore influential examples for a variety of queries. |
May 16, 2024 | We’ve open-sourced LLM Comparator, a visualization tool to help LLM developers make sense of side-by-side evaluations. Learn more in our blog post and at goo.gle/llm-comparator, or jump in and try the in-browser demo. |
projects
selected publications
- LLM Comparator: Visual Analytics for Side-by-Side Evaluation of Large Language ModelsIEEE Transactions on Visualization and Computer Graphics, 2024
- The MultiBERTs: BERT Reproductions for Robustness AnalysisICLR (spotlight), 2022
- The Language Interpretability Tool: Extensible, Interactive Visualizations and Analysis for NLP ModelsIn Proceedings of the 2020 Conference on Empirical Methods in Natural Language Processing: System Demonstrations, 2020